Science and Mathematics
Space-Filling Designs for Computer Experiments and Their Application to Big Data Research
December 14, 2021 at 4:00pm – 5:00pm EST
Virtual (See event details)
This event has already occurred. The information may no longer be valid.
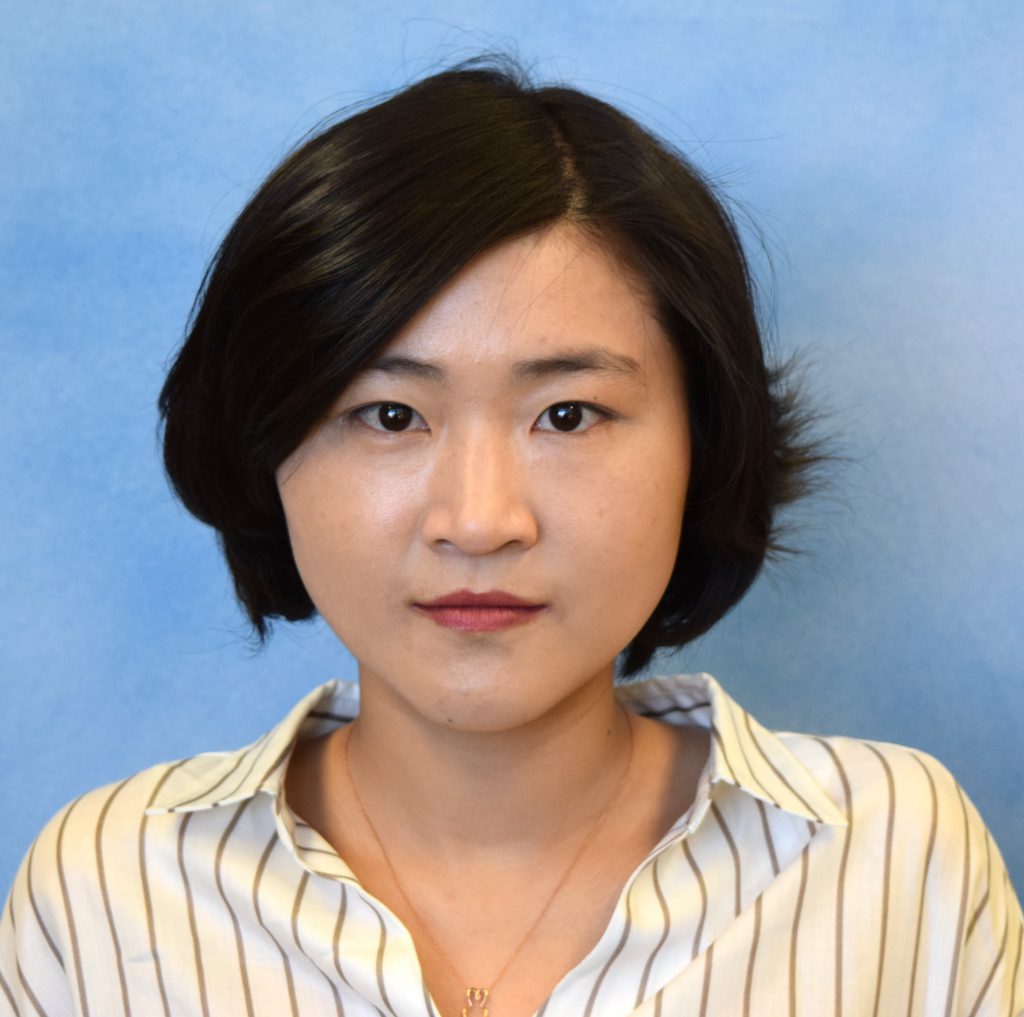
The Department of Mathematics in the College of Arts and Sciences is honored to welcome Dr. Chenlu Shi to deliver the weekly colloquium. Dr. Shi is an Assistant Adjunct Professor at UCLA, where she works in Experimental design, computer experiment, and big data reduction.
Abstract: Computer models are powerful tools used to study complex systems from almost every field in natural and social sciences. However, the complexity of the computer model results in the high computational cost for the investigation. This issue calls for computer experiments that aim at building a statistical surrogate model based on a set of data generated by running computer models. Space-filling designs are the most accepted designs for computer experiments. The first part of this talk will give a broad introduction to space-filling designs. Thanks to the guaranteed space-filling property in the low dimensional projections of the input space, a family of space-filling designs, so-called general strong orthogonal arrays, is appealing. We will present some theoretical results on general strong orthogonal arrays in the second part of the talk.
In the third part of the talk, the application of space-filling designs to big data research will be discussed. Using limited computing resources, numerous challenges arise from the process of carrying out standard statistical analysis on the large-scale dataset. One way to overcome these challenges is to conduct standard statistical analysis based on a subset of data from full data. Alternatively, in big data analysis, learning techniques have become valuable tools. But, the performance of learning algorithms relies heavily on user-set hyperparameters. In the talk, we will present a subdata selection method for big data, which makes use of the idea of space-filling designs and thus is robust to model misspecifications, and some preliminary results on hyperparameter optimization for learning techniques.
Contact Leah Quinones at Laquinon@syr.edu for zoom link
This event was published on December 10, 2021.
Event Details
- Category
- Science and Mathematics
- Type
- Talks
- Region
- Virtual
- Open to
- Public
- Cost
- Free
- Organizer
- CAS-Department of Mathematics
- Contact
- Leah Quinones
Laquinon@syr.edu
3154431471
- Accessibility
- Contact Leah Quinones to request accommodations