Science and Mathematics
Persistent Topological Descriptors for Functional Brain Network
March 24, 2021 at 11:40am – 12:50pm EDT
Virtual (See event details)
This event has already occurred. The information may no longer be valid.
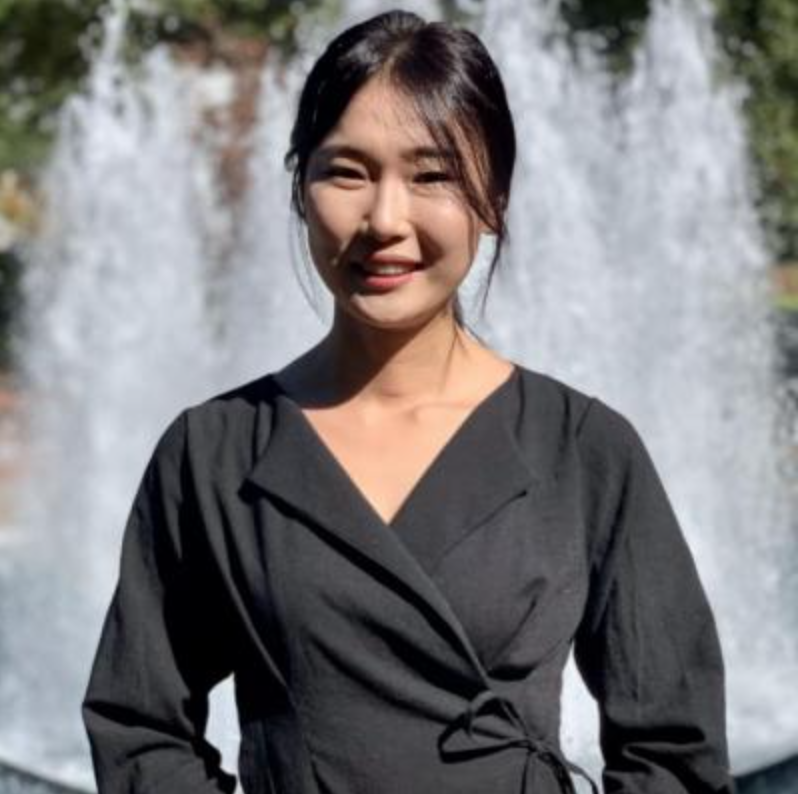
The Department of Mathematics in the College of Arts and Sciences is honored to welcome Ms. Hyunnam Ryu to deliver the weekly colloquium. Ms. Ryu is a PhD candidate in the Department of Statistics at the University of Georgia. Her research interests include topological data analysis, functional brain network analysis, and Bayesian data analysis.
Abstract: We compare the topological features of functional brain networks. In general, functional brain networks are dealt with in an elementwise manner based on the connectivity matrix as part of network data analysis. This tends to ignore the higher-order topology of the network, which can have significant implications. In recent studies, researchers have been interested in topological data analysis. Persistent homology is known to be useful for studying dynamic topological invariants hidden in complex data obtained from topological space. Analysis using persistent homology not only captures topological features that could be overlooked in the network data analysis but also addresses threshold selection problems commonly found in network data analysis.
We use persistent homology to compare the topological features of brain networks. We construct a brain network from the fMRI time series BOLD signal and calculate the persistent homology through the weighted brain network. Also, we compare the summarized topological features of different subject groups.
This event was published on March 22, 2021.
Event Details
- Category
- Science and Mathematics
- Type
- Talks
- Region
- Virtual
- Open to
- Public
- Cost
- Free
- Organizer
- CAS-Department of Mathematics
- Contact
- Leah Quinones
laquinon@syr.edu
3154431471
- Accessibility
- Contact Leah Quinones to request accommodations